November 23, 2023
The Role of Predictive Models in the Future of Underwriting
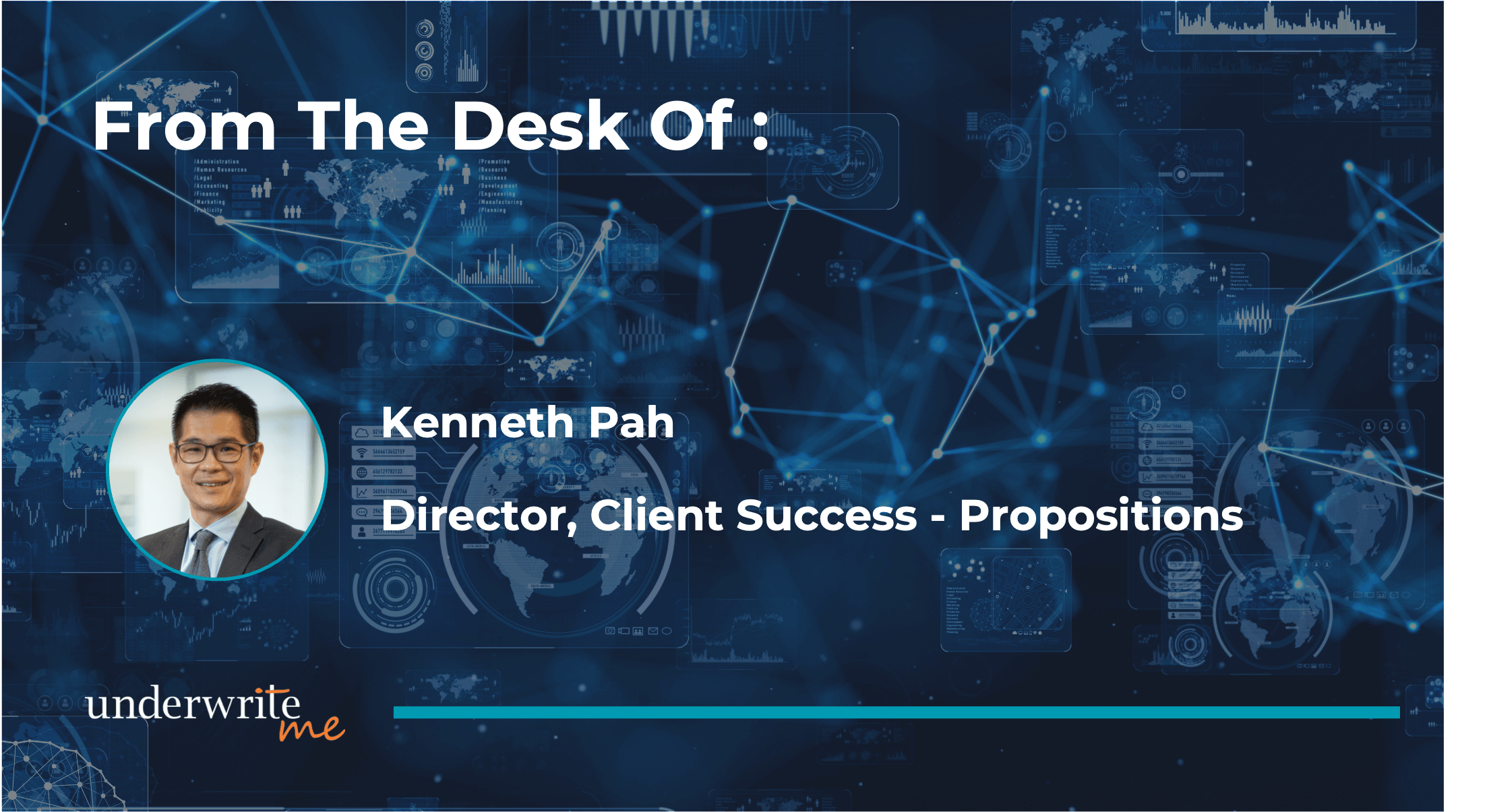
In the earlier article on Innovation, Stephen Connolly challenged insurers to think BIG! That material growth can only be achieved through a reframe in mindset and not only to think about existing process optimisation but critically, innovation aimed at transforming the customer journey, and at a broader scale – helping to make insurance more accessible across all market segments.
At the core of predictive models is its ability to analyse vast datasets of traditional and non-traditional sources to identify patterns and correlations which would otherwise not have been identifiable by the human underwriter.
As the pace of digitisation and digitalisation continues to accelerate, so has the quantum of data available for use as model inputs. An analysis across some of our implementations revealed a 167-fold increase in newly available structured data on health disclosure that can be used to provide business insight as well as inputs into predictive models.
With predictive models, insurers are now also able to unlock value from non-traditional data sources such as banking transactions and mobile data for automated decision making.
In constructing predictive models, machine learning techniques use algorithms to train a model, identifying patterns within datasets. Subsequently, the trained model generates predictive scores, which are then fed
As we look towards operationalising predictive models, we observe that the best outcomes come from combining predictive models and rules-based logic. This is due to the multi-faceted nature of risk assessments, where some risk criteria are better assessed through rule logic while others through predictive models. Striking the optimal balance involves considering the nature of the assessment as well as the type and volume of data available to inform the decision-making process.
At UnderwriteMe, we work with insurers to realise this Future of Underwriting through our technology platform, the Decision Platform, which enables the integration of predictive models as well as external data sources with the underwriting engine.
Figure 1: The Decision Platform is a modular platform aimed at enabling optimised decision-making capability, leveraging both internal and external data sources.
With more than one decision-making module at play, orchestration capability now becomes essential for determining the manner of interaction (i.e., sequencing) between two (or more) decision-making components such as the underwriting engine and predictive model(s). This orchestration functionality is administered through the Decision Studio module within the Decision Platform.
Unlike the underwriting engine, different predictive models would be evoked at different points in time to cater for different scenarios.
Predictive model outputs (e.g., scores) are integrated into the underwriting engine as configurable attributes that can be identified, recorded, and applied across different scenarios. This can be in the form of contributions to co-morbidity ratings and/or risk factors through a scoring system.
We see the use of predictive underwriting models falling into two primary areas.
Firstly, segmentation models are used to categorise customers into different risk pools, enabling insurers to vary the number and type of underwriting questions and medical requirements across different segments.
As regards question variation, while much of the narrative today revolves around which question set is to be used for a given model score (i.e., a longer or shorter question set), we see the opportunity for predictive models to enable question-specific determination, thus taking the level of personalisation to a new level.
Secondly, from an automation optimisation perspective, we work with insurers and the team at Pacific Life Re in developing predictive underwriting models to improve and simplify the underwriting process when an instant decision cannot be attained through rule logic alone.
With that said, the application of predictive models yet to be operated at scale across the industry, primarily due to the knowledge and expertise required for the creation and management of predictive models. There is a high dependency on subject matter experts, like data scientists, data engineers and software developers.
While there is broad agreement that the Future of Underwriting involves a blend of predictive models, rule logic and various data sources – both internal and external to insurers, the challenge lies in seamlessly connecting all these elements to deliver instant, personalised, and low-effort customer journeys.
At UnderwriteMe, we continue to help insurers realise this Future of Underwriting and in doing so, help make insurance more accessible for everyone.